OctoML’s new features turn AI/ML models into software functions
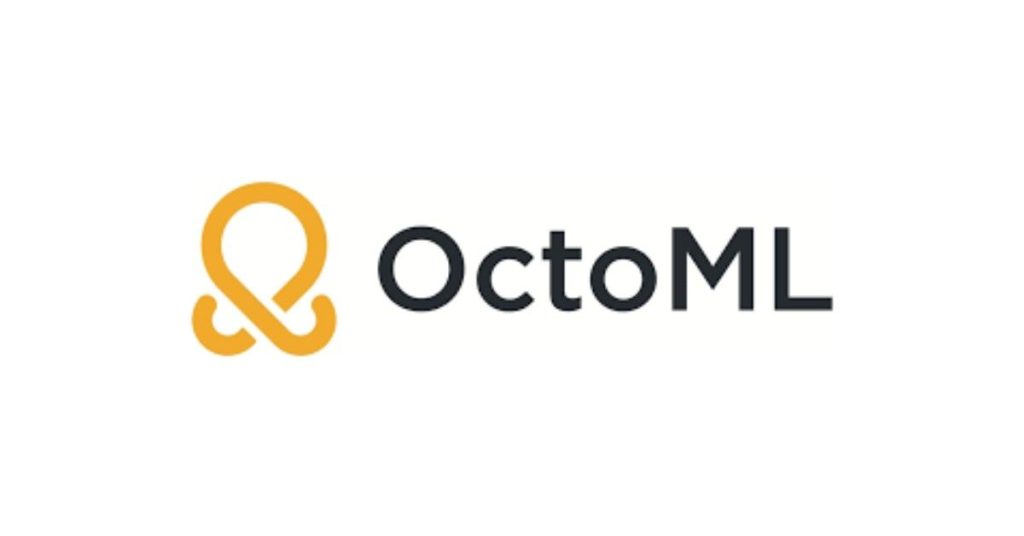
OctoML is expanding its platform with the addition of DevOps capabilities which the company says will accelerate the development of AI applications by reducing bottlenecks in production deployment. The new platform release will allow developers and IT teams to transform AI models into software functions that can be integrated into the existing application stacks and DevOps workflows.
OctoML’s new DevOps capabilities turn AI-trained models into models-as-functions to run from cloud to edge independent of what hardware infrastructure is underneath. OctoML claims that 47% of trained ML models do not reach the production stage, while the rest of the models take an average of 12 weeks to be deployed. The challenges faced by IT teams include the dependencies between ML training framework, model type, and the compatible hardware. The new OctoML platform will be a way to abstract out these complexities and enable production-ready software functions.
“Our new solution is enabling them to work with models like the rest of their application stack, using their own DevOps workflows and tools,” said Luis Ceze, CEO at OctoML. “We aim to do that by giving customers the ability to transform models into performant, portable functions that can run on any hardware.”
Some of the key highlights of the platform include the automatic detection of dependencies and resolving them to optimize model code and accelerate model deployment for any hardware. The OctoML provides more than 80 deployment targets in the cloud and at the edge with accelerated computing. The expansion in the software catalog will cover several ML frameworks and acceleration engines, such as Apache TVM, and software stacks from chip manufacturers.
OctoML also announced that the Nvidia Triton inference software solution will be integrated with the OctoML platform for model-as-a-functions. The integration of Nvidia Triton will provide users with the access to choose, integrate, and deploy Triton-powered inference from any framework on data center servers.
“Nvidia Triton is the top choice for AI inference and model deployment for workloads of any size, across all major industries worldwide,” said Shankar Chandrasekaran, product marketing manager at Nvidia. “Its portability, versatility and flexibility make it an ideal companion for the OctoML platform.”
Recommended reading: Nvidia renews efforts in Edge AI for smart cities with new solutions and partnerships
“Nvidia Triton enables users to leverage all major deep learning frameworks and acceleration technologies across both GPUs and CPUs,” said Jared Roesch, CTO at OctoML. “The OctoML workflow extends the user value of Triton-based deployments by seamlessly integrating OctoML acceleration technology, allowing you to get the most out of both the serving and model layers.”
JFrog recently announced the commercial availability of JFrog Connect, a DevOps platform that will integrate into the JFrog platform. JFrog DevOps capabilities will allow enterprises to effectively manage thousands of edge IoT devices through operational models and interactive UI across cloud, on-premise networks, and multi-cloud deployments.
Comments