Summit gathering explores edge computing, AI for vision
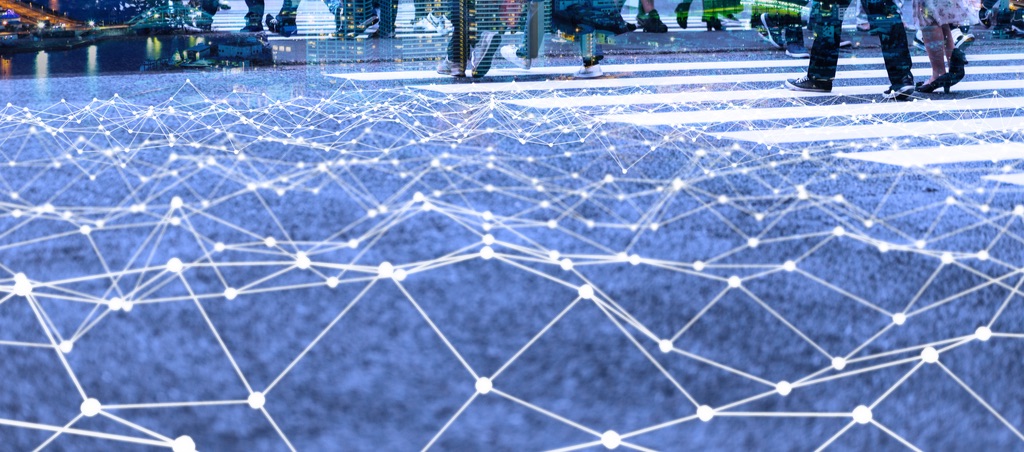
The field of computer vision has been changing at a rapid pace in 2020. It has been accelerated by both numerous advances in chip technology and a pressing market need for health and safety solutions. A summit gathering held by the Edge AI and Vision Alliance has been gathering many of the big industry visionaries, so to speak, of AI and vision systems to continue to advance the science and the market for edge AI.
The 2020 Embedded Vision Summit started with a keynote from UC Berkeley professor David Patterson on the trend towards domain-specific architectures for chips and how these architectures efficiently run AI workloads. Patterson is a co-inventor of the RISC (reduced instruction set computing) architecture used today in chips by ARM and is also a contributor to Google’s Tensor Processing Unit (TPU) chip used for running AI workloads in data centers.
Jeff Bier, industry consultant and founder of the Edge AI and Vision Alliance, wrote that advancements are coming fast and furious due to five key requirements. In an article for EE Times, he noted that bandwidth, latency, economics, reliability, and privacy have been pushing AI to the use of edge computing.
Bandwidth and latency have been two common requirements across edge computing use cases, but even more so in vision systems. The amount of data coming from multiple video feeds in a surveillance system can overwhelm the internet connections used to send data to the central cloud for processing. Likewise, in other cases it takes too long for a system to respond to sensory input, citing the off used example of self-driving cars needed to respond immediately to the presence of a pedestrian. The car’s computer has a few hundred milliseconds to act, Bier writes. There isn’t enough time to send images to a central cloud for processing in this case.
Both bandwidth and latency requirements have an impact on the economics of where and how to perform AI on datasets. Edge computing can reduce the amount of data that is sent to the cloud, resulting in a more economical solution, according to Bier.
The localization of data is a key theme as well. For reliability and privacy, the localization of data enables information to be processed in the absence of an internet connection (in case of connectivity is lost during a storm, for instance). The other advantage: sending less data (or in the case of some new chip designs, no data) to the cloud reduces issues with personal data getting into the wrong hands.
While some of the Summit sessions have taken place, the event continues on September 22 and 24 with more presentations from researchers and industry leaders. LG Electronics, Algolux, Synopsys, Zebra Technologies, Perceive, Inc., and others will present on edge AI implementation. Meanwhile, well over 30 companies will exhibit edge AI chips and software solutions during the virtual event, including CEVA, Cadence, Hailo, Intel, Lattice, Nvidia, Perceive, Qualcomm, and Xilinx. For example, BrainChip announced that it is exhibiting a new generation of edge AI chips, dubbed Akida. The chip is billed as a Neuromorphic System-on-Chip (NSoC) offering advanced neural networking capabilities in a small, ultra-low power form factor.
Market research company Omdia forecasts that global AI edge chipset revenue will grow from $7.7 billion in 2019 to $51.9 billion by 2025. Edge inference has emerged as a key workload and many companies have introduced chipset solutions to accelerate AI workloads.
This interview was originally posted on Biometric Update.
Article Topics
bandwidth | chips | computer vision | edge AI | Edge AI and Vision Alliance | edge computing | latency | market research
Comments