Study says AI and Google edge computing can detect disease in real-time
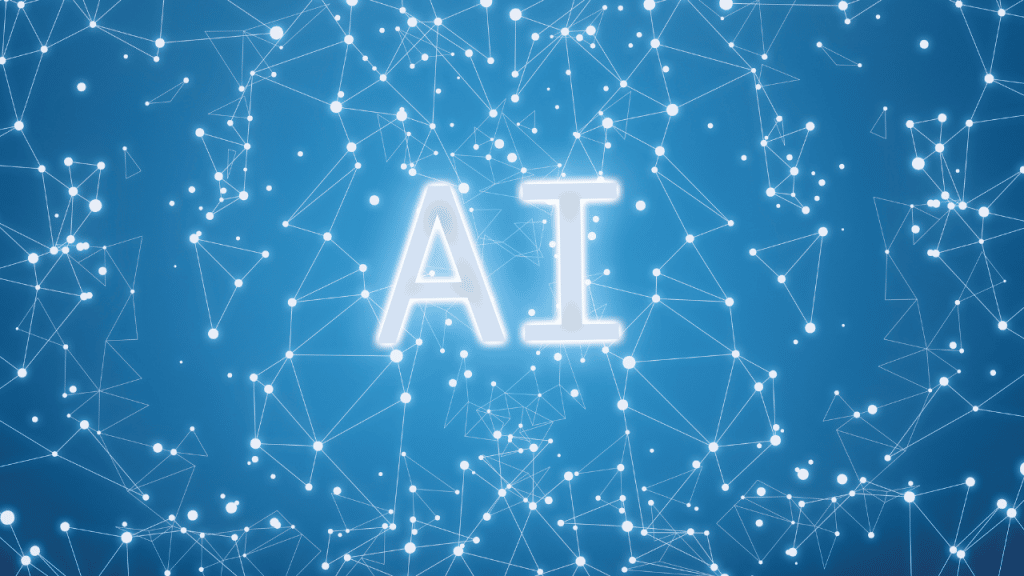
Machine learning implemented at the edge has attracted significant attention in medicine and healthcare. Researchers now say advancements in edge computing and machine learning models will enable better detection of pathogens, and aid in disease control.
The University of California, Santa Cruz conducted a study showing how Google’s edge computing platform, coupled with machine learning, can detect pathogens in real-time.
Accurate diagnosis at the point of use depends on the ability to detect and identify multiple biomarkers simultaneously in real time. However, researchers say detecting numerous biomarkers can be challenging as their signals may vary in shape and quality due to varying factors.
Compared to the traditional shift-and-multiply method for multiplexed single-molecule identification, the study says the proposed deep neural network solution provides a 40% increase in classification accuracy. Machine learning algorithms, particularly the deep neural network approach, are highly effective in accurately identifying and classifying fluorescence signals.
The study also reveals that smaller edge devices like the Google Coral Dev Board can perform complex computational tasks like high-resolution multi-scale event detection and classification. This is made possible using deep neural network models with a few thousand parameters. Endpoint near-sensor data analysis has several advantages, including decreased energy consumption and reduced network load in endpoint-cloud communication.
“The Coral Dev board is not capable of training the model and requires a desktop computer for training and model transfer steps. We believe this limitation can be solved with future updates of the hardware and libraries or by utilizing a cloud-based server for this specific task,” the researchers add.
The proposed model combines fast wavelet particle detection, short-time Fourier Transform analysis, and deep neural network identification to achieve high accuracy. The study involved conducting experiments that used multi-spot optical excitation to target the nucleic acids of Klebsiella Pneumoniae bacteria, which are associated with pneumonia in certain patient populations. Fluorescence signals with different characteristics like amplitude, duration, and quality were generated on an optofluidic waveguide chip during the experiments.
The study shows that this combination can accurately achieve real-time disease detection, resulting in a 99.8 percent accuracy rate.
Article Topics
AI/ML | disease control | DNN | Google | inferencing | IoT | model training | neural network | sensor | U.C. Santa Cruz
Comments