NTT, MIT Researchers develop technique for efficient AI on IoT edge devices
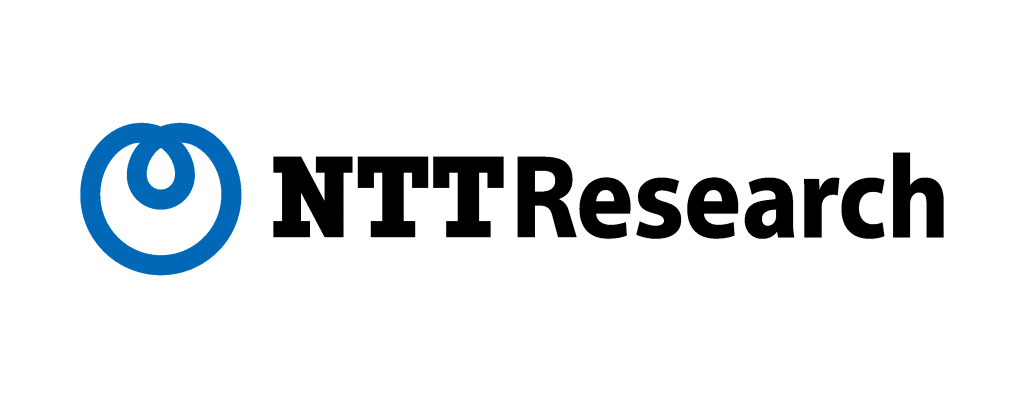
A group of scientists, including researchers from NTT Research, MIT and the Physics and Informatics (PHI) Lab, recently demonstrated a new technique called Netcast. This technique uses optical technology to run deep neural networks (DNNs) efficiently, and it can enable advanced DNNs on resource-constrained Internet of Things (IoT) edge devices.
Deep neural networks are a type of machine learning and artificial intelligence used to analyze and interpret large amounts of data quickly and accurately. They are used in various applications, including image and speech recognition and natural language processing. DNNs require immense amounts of training data and processing power to operate effectively.
The researchers state that energy consumption is still a persistent issue in matrix algebra used by DNNs, despite recent advancements in analog techniques such as neuromorphic, analog memory and photonic meshes. The researchers suggest that Netcast can solve the problem of weight memory access on edge devices. This would lead to a decrease in both latency and energy consumption related to matrix algebra.
Previous research revealed that relying on cloud servers to handle computational power for DNN inference may cause delays and security concerns, such as communication channel breaches.
Dr. Ryan Hamerly, a contributor to this research and lead author of a 2019 paper on optical neural networks and photoelectric multiplication, offered a solution to this challenge. He proposed a solution that involved encoding the DNN model in an optical signal and transmitting it to an edge processor.
The team used Dr. Hamerly’s proposal to develop Netcast. It uses a photonic edge computing architecture with a smart transceiver that can integrate into cloud computing infrastructure. Further, it also has a time-integrating optical receiver for the client’s end.
The MIT researchers showcased Netcast using a smart transceiver and a client receiver connected by 86 km of optical fiber in Boston. They claimed Netcast could achieve image recognition accuracy of up to 98.8 and reduce optical energy consumption to less than one photon per MAC.
“The difference is that a GPU high-bandwidth memory link consumes around 100 watts and only connects to a single GPU, whereas a Netcast link can consume milliwatts, and by using trivial optical fan-out, one server can deploy a DNN model to many edge clients simultaneously,” says Dr. Ryan Hamerly.
Article Topics
AI/ML | deep learning | edge AI | energy efficiency | IoT | MIT | NTT
Comments