HPE Swarm Learning uses blockchain, edge data to create privacy-preserving ML solution
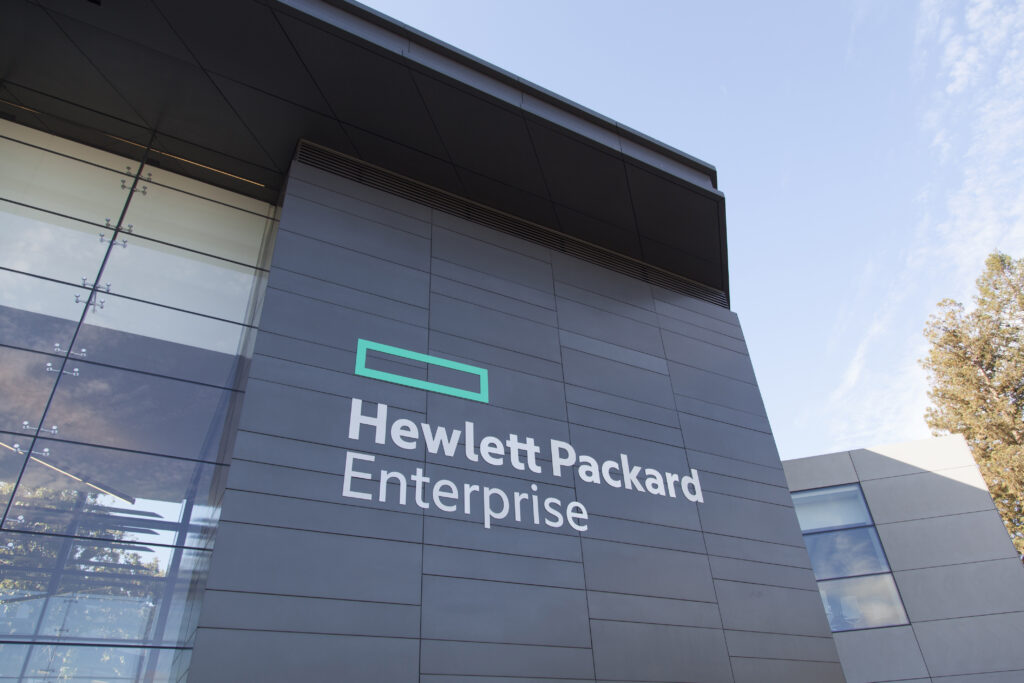
Hewlett Packard Enterprise has announced the launch of HPE Swarm Learning, a privacy-preserving framework to increase accuracy and reduce bias in AI models. As a decentralized machine learning solution, the framework enables the data to stay in local servers and only share the learnings to improve models by reducing bias. Keeping data local also has the benefit of enhancing data privacy, data ownership, and efficiency concerns.
Swarm learning is a decentralized machine learning infrastructure built on blockchain technology to enable enterprise customers to efficiently use the power of distributed data while protecting data privacy and security. In general, swarm learning leverages the compute power at the data source (for example, at the edge where it is created) and ensures security through “tested” blockchain technology.
As a decentralized solution, the privacy-preserving framework gives data owners better control over access to sensitive information and extracts insights from the source data without exploiting data privacy. The built-in blockchain technology provides the capability to share the learnings of the models with participating ML nodes in the HPE Swarm Learning peer network for insights.
“HPE is contributing to the swarm learning movement in a meaningful way by delivering an enterprise-class solution that uniquely enables organizations to collaborate, innovate, and accelerate the power of AI models while preserving each organization’s ethics, data privacy, and governance standards,” says Justin Hotard, executive vice president, and general manager, HPC & AI, at HPE.
Overall, the HPE Swarm Learning framework fully supports containerized software with Swarm Operator for command-line management, and swarm learning for running user-defined machine learning algorithms. It also comes with a blockchain-based smart contract between participants to provide attacks on the machine learning models while merging.
Some of the use cases of early HPE Swarm Learning adopters include the University of Aachen, which studies histopathology to accelerate the diagnosis of colon cancer, and TigerGraph’s anomaly detector, which is used to help banks prevent credit card fraud. In the former, the researchers trained AI models using the HPE Swarm Learning framework on three groups of patents from Ireland, Germany, and the U.S. and validated the performance in two datasets using the same swarm learning-based AI models. “The results demonstrated that the original AI models, training only on local data, were outperformed using swarm learning due to sharing learnings, but not the patient data, with other entities to improve predictions,” the researchers note.
“Swarm learning is a new, powerful approach to AI that has already made progress in addressing global challenges such as advancing patient healthcare and improving anomaly detection that aid efforts in fraud detection and predictive maintenance,” Hotard further added.
Hewlett Packard Enterprise also announced the release of the HPE Machine Learning Development System, which aims to reduce entry barriers for enterprise customers while building and training machine learning models at scale.
Article Topics
AI/ML | blockchain | data sovereignty | edge data | HPE | machine learning | privacy
Comments