Generative AI: What it is and how it’s shaping edge infrastructure
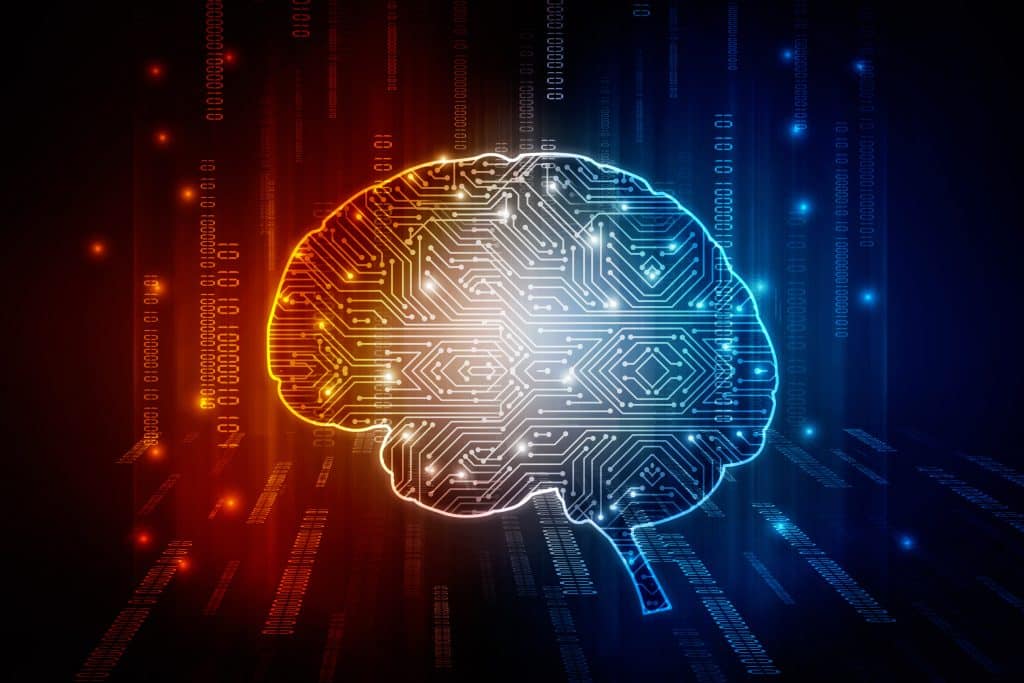
There have been extensive technological advancements in the field of artificial intelligence that have revolutionized the world’s interaction with digital systems that surround us. In particular, generative artificial intelligence (AI) is being hailed as a game-changer technology with the potential to bring stagnating productivity levels and ignite a new wave of scientific innovation.
According to Bloomberg Intelligence, generative AI is anticipated to grow at a CAGR of 42 percent over the coming decade. From embedded hardware manufacturers to AI software startups, technology companies are aiming to leverage the immense potential of this generative AI landscape, expecting a transformative impact on the entire technology sector.
In simpler terms, generative AI utilizes written prompts to create new content, code, images, text, and videos. This technology relies on neural networks to identify patterns and structures within existing data, enabling it to generate entirely new and original materials.
The development of generative AI has undergone various iterations since the 1950s, but a significant breakthrough occurred with the introduction of the transformer architecture, initially designed for natural language processing. The transformer model is a type of neural network that acquires context and meaning by tracking relationships within sequential data, such as the words in this sentence.
Having undergone pretraining on vast datasets to learn complex patterns and relationships, these large language models (LLMs) have acquired the ability to mimic human language processing. These LLMs serve as the foundation for generative AI, being neural networks capable of comprehending and analyzing natural language.
While generative AI has found applications in almost every industry, many are now exploring the role of edge computing in supporting generative AI and how manufacturers of embedded devices can align their design objectives to cater to this expanding market.
Intersection of edge computing and generative AI
The Hardware, AI, and Neural-nets (HAN) Lab at the Massachusetts Institute of Technology has introduced TinyChat, a system designed for deploying large language models at the edge. In a demonstration, the Llama-2 model was showcased running at a rate of 30 tokens per second on Nvidia Jetson Orin hardware, with the capability to support various models and hardware configurations.
When we consider the significance of deploying large language models at the edge, it becomes evident that many embedded applications, spanning from automotive to industrial manufacturing, necessitate instantaneous access and service without depending on a stable internet connection. Bringing large language models to the edge offers the advantage of mitigating the inconveniences associated with delays commonly encountered when relying on cloud services.
[Note: Not all generative AI tools are built on LLMs, but all LLMs are a form of generative AI.]
In today’s context, the deployment of edge computing devices in remote areas, particularly in industries such as oil and gas, has the potential to simplify the monitoring and management of entire infrastructures. This is achieved by leveraging insights generated by large language models at the edge to provide real-time analysis of critical data. Implementing large language models at the edge can help businesses mitigate concerns related to network outages and also eliminate the expenses associated with transferring data from an edge location to the cloud.
The industry recognizes a strong connection between generative AI technology and edge computing, as well as the Internet of Things (IoT). Through generative AI, businesses can enhance their ability to predict future events and trends with greater precision, thereby improving the intelligence level and production efficiency of edge devices. In this context, it is essential for generative AI to conduct model training and inference directly on edge devices.
Generative AI and the future of edge infrastructure
It might not be completely wrong to say that generative AI is shaping the future of edge infrastructure. While most of the edge applications today are bringing operational efficiency without the use of generative AI. But it is not far from when we see the use of large language models and generative AI bringing revolutionary changes to the way we see edge applications today.
While embedded hardware manufacturers are already working on implementing large language models on edge devices, all of this is to support generative AI technology to support the wide range of edge applications in mission critical industries.
Edge AI chip provider Quadric has recently unveiled its latest development – compatibility between its general-purpose neural processing unit IP core, Chimera, and the Llama 2 model. This enhancement positions the company as a standout player in the landscape of chip providers, thanks to its support for large language models.
These developments highlight the growing trend of embedded hardware manufacturers increasing their investment in generative AI. As a result, we can anticipate a proliferation of large language model implementations on edge devices in the near future.
Article Topics
edge AI | edge applications | edge computing | generative AI | LLMs
Comments