Avoid unnecessary science experiments by using the ‘Five Principles of Edge AI’
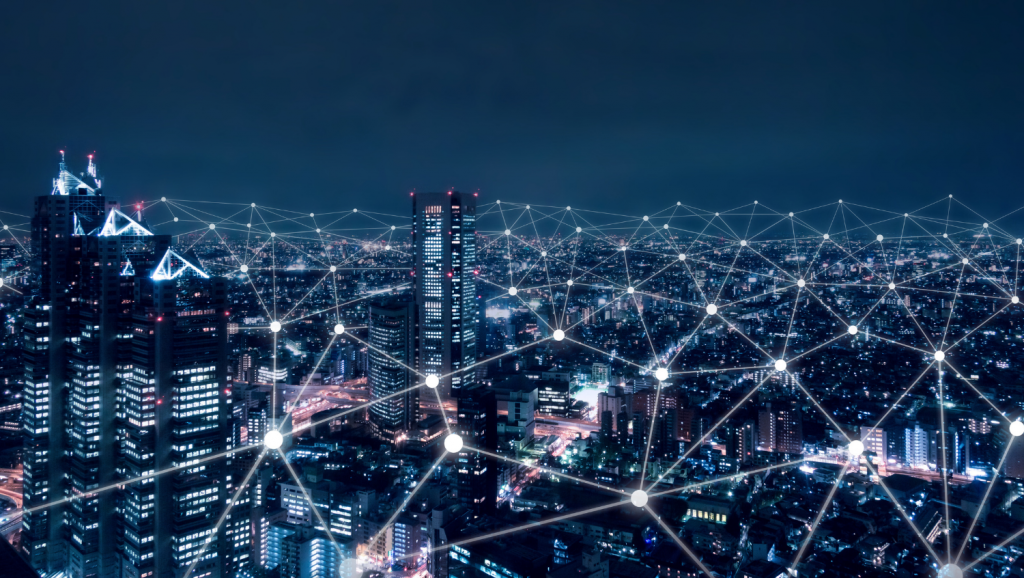
This is a guest post by Eric Simone, CEO of Clearblade.
Artificial Intelligence (AI) and edge computing are two technologies that offer amazing potential and are consistently in the technology headlines. Together they promise to transform the way we run our businesses and automate decisions. Every day, new AI and edge companies emerge making bold promises that will change the world. News stories offer amazing glimpses into our future with devices and interactive displays like the ones featured in Westworld. These visions of the future are interesting to think about; futurists like Michio Kaku are amazing thought leaders that inspire us to think about these possibilities. But as a CEO of a software company living in the real world, I must make sure we deliver on promises that deliver business results today.
So how do we separate fact from fiction, marketing hype from business reality? Sadly, many AI and Edge initiatives fail after huge investments. These edge AI projects may be using datasets that are insufficient, algorithms that never actually learn, or are focused on business problems delivering no real ROI. To help you on your edge AI journey there are critical features to focus on to uncover the truth and make the right decisions for your company.
When it comes to AI, separating technology from data science and domain expertise is extremely difficult to do, especially with all of the noise in the current marketplace. To filter that noise, it’s helpful to break down an AI project into its most basic tasks.
AI data acquisition
First, we need to train the AI model with machine data, so we need to acquire a large dataset that includes as much current information as possible. This requires domain experts – people that understand the business of what we are trying to automate, such as an experienced operational engineer on the oil rig, on the auto plant floor, or at the airport maintenance facility.
AI algorithm selection
Here we look at the input data we have as features and try different mathematical algorithms that will provide us with the correct answers most often. This activity takes a data scientist that understands a particular industry such as oil & gas, car manufacturing, or airplane maintenance.
AI Model Training
Now we pump the data through the algorithm to train the AI model. This model runs in real-time and adapts, eventually generating the most accurate answers and behavior. This activity requires both the domain expert and data scientist working together to validate the behavior.
Next step: moving to the edge
The edge industry is similarly confusing because the definition of “edge” depends on your frame of reference. Are we focusing on edge hardware, network, software, or applications? To separate the noise and finish our edge AI work by selecting the edge hardware, AI model, and then move to deployment.
Edge hardware selection
The edge industry is similarly confusing because the definition of “edge” depends on your frame of reference. Are we focusing on edge hardware, network, software, or applications? To separate the noise and finish our edge AI work by selecting edge hardware, which in this case can be defined as a compute device that does not reside in the cloud or the data center. This is typically a gateway with enough computing power, storage, and connectivity for running the AI model.
Edge AI model deployment
Now let’s deploy the model and algorithm and distribute it to our physical Edge so it’s running as close to where the data is being generated as possible. This model will continue to be updated as the model improves.
Edge Application Implementation
The final step is to build an application around the data inputs with various connectivity protocols (BLE, LoRaWAN, MODBUS, CANBUS, etc.) and process it through our AI algorithm to make decisions to drive automation into our machines.
Five Principles of Edge AI
In 30-plus years of being a software developer, CTO, and now CEO of a leading-edge software company, I have never seen such excitement and promise, but to be successful we must sift through all the AI and Edge “noise” to reduce confusion to save time and money.
To move beyond the hype, we must build an edge AI foundation on five core technology principles to make sure the technologies we have chosen are viable:
– The Edge AI infrastructure must have access to the necessary machine data to train and then run against in real time.
– The Edge AI software must be able to run the AI model that was created by our business domain knowledgeable engineers and data scientists.
– The Edge AI hardware must be powerful enough to run this algorithm as close to where the machine data is being generated.
– The Edge AI software must be open enough to adjust to different machine protocols without additional expense or engineering.
– The Edge AI solution must be able to scale to and easily manage thousands of unique instances across the enterprise.
Of course, there are challenges beyond data science and technology. This includes understanding your business and making sure you measure your results to validate the investment. The obvious critical measurement is business value and a clear ROI—one that makes sure you are not taking years to deliver value.
To date, I have witnessed far too many failed edge AI “science experiments.” Ensure your projects do not include expensive bespoke software development and instead make sure they focus on domain specific, market differentiating expertise related to your industry. Only by leveraging proven edge and AI technology and focusing on your business can you ensure that your edge AI projects are successful.
About the author
Eric Simone is CEO of Clearblade. Clearblade provides edge computing software that lets businesses securely run and scale IoT devices in real-time. The company’s IoT platform helps companies synchronize, manage and deploy IoT systems with one common stack so developers can build in the cloud and push to the edge.
DISCLAIMER: Guest posts are submitted content. The views expressed in this blog are that of the author, and don’t necessarily reflect the views of Edge Industry Review (EdgeIR.com).
AWS rolls out second Local Zone edge node in Los Angeles
Article Topics
AI | application orchestration | artificial intelligence | ClearBlade | data analytics | edge AI | edge applications | edge computing | edge hardware | edge infrastructure | IIoT
Comments