Akamai, Neural Magic collaborate to improve deep learning capabilities on distributed computing infrastructure
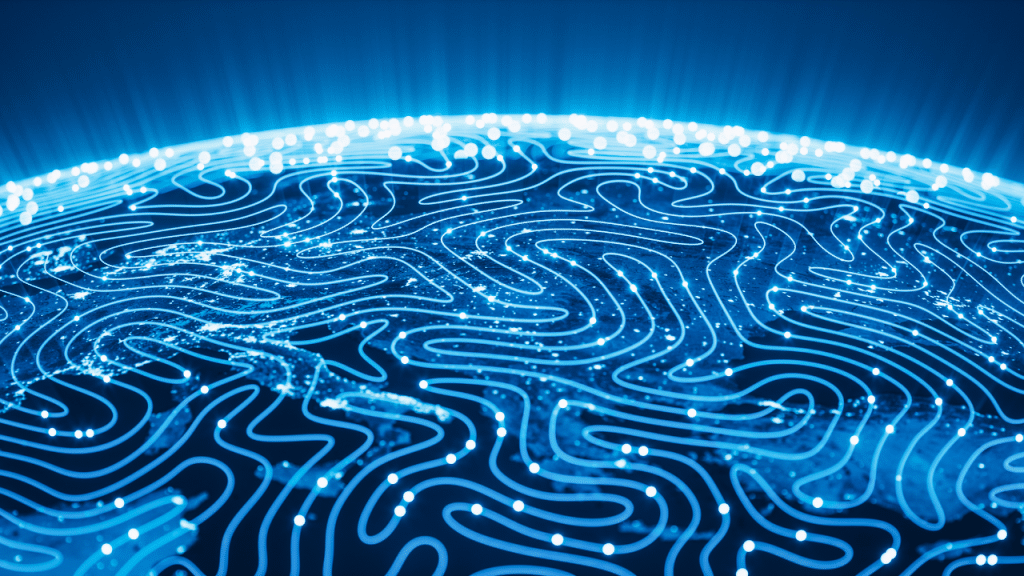
Akamai Technologies, a provider of cloud and edge solutions, has partnered with Neural Magic, a company specializing in AI software solutions. The collaboration aims to enhance Akamai’s distributed computing infrastructure with Neural Magic’s AI acceleration software, which focuses on optimizing the use of CPUs instead of GPUs.
Through the integration of Neural Magic’s software, the partnership aims to implement these capabilities on a global scale. Such a development would enable enterprises to execute data-intensive AI applications with reduced latency and enhanced performance, regardless of their physical location.
“Specialized or expensive hardware and associated power and delivery requirements are not always available or feasible, leaving organizations to effectively miss out on leveraging the benefits of running AI inference at the edge,” says John O’Hara, senior vice president of Engineering and COO at Neural Magic.
Neural Magic uses automated model sparsification and CPU inference to efficiently implement AI models on CPU-based servers. When combined with Akamai’s capabilities, this technology is particularly advantageous for edge computing applications, where data is processed in close proximity to its source.
Furthermore, Akamai’s recently launched Generalized Edge Compute (Gecko) initiative seeks to enhance cloud computing capabilities within its extensive edge network. According to Dr. Tom Leighton, Akamai’s co-founder and CEO, “Gecko represents the most significant advancement in cloud technology in a decade.”
“Scaling Neural Magic’s unique capabilities to run deep learning inference models across Akamai gives organizations access to much-needed cost efficiencies and higher performance as they move swiftly to adopt AI applications,” says Ramanath Iyer, chief strategist at Akamai.
A research paper on the support for Llama 2 in DeepSparse was recently published by Neural Magic. According to the company, this paper shows that applying model compression techniques like pruning and quantization during the fine-tuning process can lead to a compressed version of the model without any loss of accuracy.
Neural Magic also claims that deploying the compressed model with their optimized inference runtime, DeepSparse, can speed up inference by up to 7 times over the unoptimized baseline, and make CPUs a viable deployment target for LLMs.
Article Topics
AI software | Akamai | deep learning | distributed computing | Neural Magic
Comments