Premio’s new whitepaper showcases the benefits of M.2 accelerators for edge AI performance
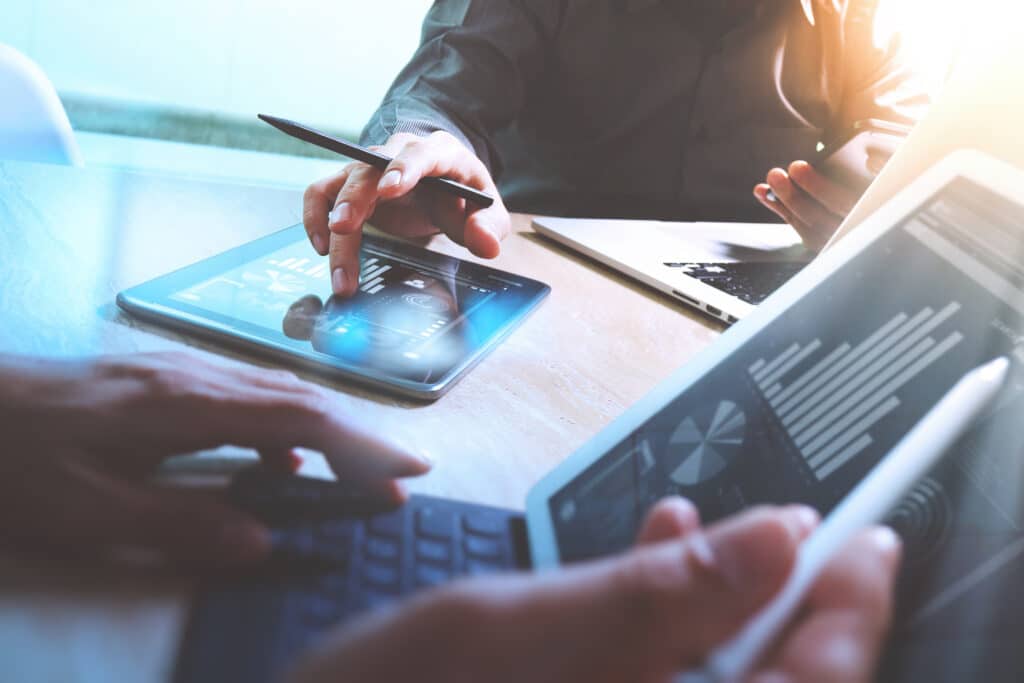
Premio Inc, a rugged edge and embedded computing technology provider, released a technical whitepaper that benchmarked the critical benefits around hardware acceleration for workloads powered with edge AI.
This whitepaper provides a comprehensive overview of M.2 domain-specific architectures and how they can improve performance for machine learning workloads in Edge AI applications.
Data growth and the need for real-time analytics are driving the AI computing framework away from general CPU/GPU options and toward specialized accelerators based on domain-specific architectures that use the common M.2 standard, according to Dustin Seetoo, Premio’s product marketing director.
Seetoo said that some of the latest AI modules hitting the market today are highly beneficial for fanless edge computers because they are smaller and even more power-efficient than traditional options.
According to Premio, this is where M.2 form-factor accelerators come in handy, as they can break down performance barriers in data-intensive apps. M.2 accelerators are a powerful design option that offers domain-specific value to system architects, allowing them to match the requirements of AI workloads exactly. Compared to a similar system using CPU/GPU technologies, an M.2-based system may more swiftly and efficiently manage inference models.
The Hailo-8 processor, according to the whitepaper, is a small edge AI accelerator that can process up to 26 tera operations per second (TOPS) and consumes only 2.5 watts of power. The Hailo-8 module can be used in edge AI deployments with an industrial-grade Premio inference computer to process object detection workloads and inference analysis quickly and accurately.
“There is a clear differentiation between a general-purpose embedded computer and one that’s designed to balance inferencing algorithms across compute, storage and connectivity,” Seetoo also said. “All these factors are necessary to effectively consolidate workload close to the point of data generation, even in rugged settings where environmental challenges are detrimental to system performance.
Edge Woman of the Year Award Interviews: Amanda Marrs, AMP Robotics
Comments