Elemeno AI’s ML-OPs platform available to help enterprises use edge data
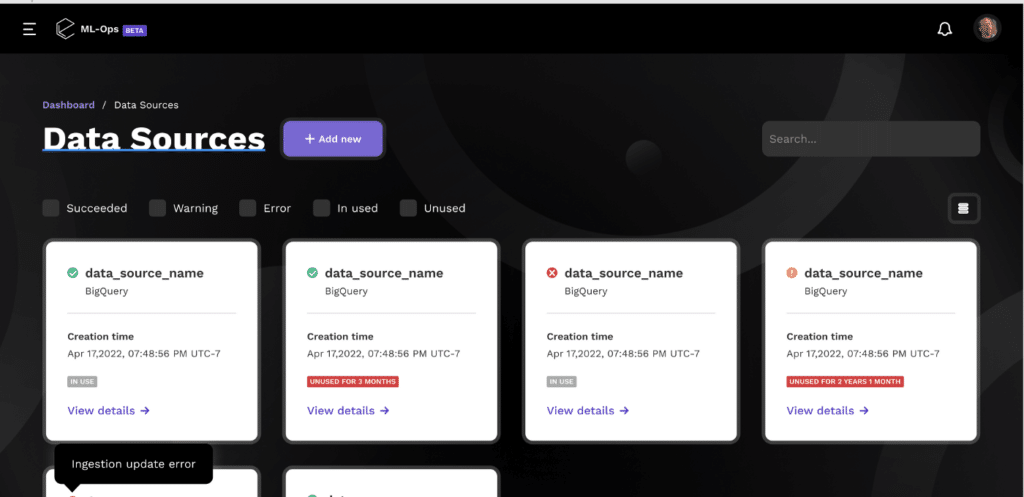
Elemeno AI, a cloud-based machine learning platform provider, has launched the first phase of its machine learning operations platform to help enterprise customers leverage advancements in artificial intelligence. The company’s SaaS “ML-Ops” platform offers an enhanced user experience for data scientists to build ML models from start to deployment. With the simplified interface, the platform aims to reduce the traditional engineering hurdles in developing and deploying machine learning models — an important step as companies deal with increasing amounts of data from edge computing systems and sensors.
The platform can connect data sources such as AWS Redshift and Google BigQuery, after which the company can configure feature ingestion pipelines. In this stage, the companies can modify the features and customize them to the specific AI application. The SaaS ML-Ops platform also manages the storage by ensuring the recent AI models are available in the deployed applications. The base model data is stored in cold storage.
“Launching our public beta means getting one step closer to solving the intricacies that are causing companies to adopt AI slowly,” says Lucas Bonatto, the CEO of Elemeno AI. Bonatto noted the gap between global adoption and investment levels highlighted in the Stanford AI Index Report of 2022, and adds “Elemeno ML-Ops is the first step to solving this problem.”
By using Elemeno AI’s no-code solutions, enterprise companies can choose between the machine learning frameworks supported by the SaaS ML-Ops platform, the company says. Some of the supported frameworks include TensorFlow, PyTorch, Scikit Learn, Keras, ONNX, and TensorFlow Lite.
Elemeno’s AI SaaS ML-Ops platform is a scalable infrastructure built on a standard serverless architecture, enabling companies to take the benefits of using Kubernetes, Kubeflow, Jupyter, and much more without being an expert.
“With ML becoming widespread, the platform tackles prominent implementation challenges, including the lack of end-to-end solutions that allow companies to manage the complete lifecycle of their ML models,” the company concludes.
Article Topics
AL/ML | data management | Elemeno AI | ML Ops | model management
Comments