Edge, edge, everywhere an edge
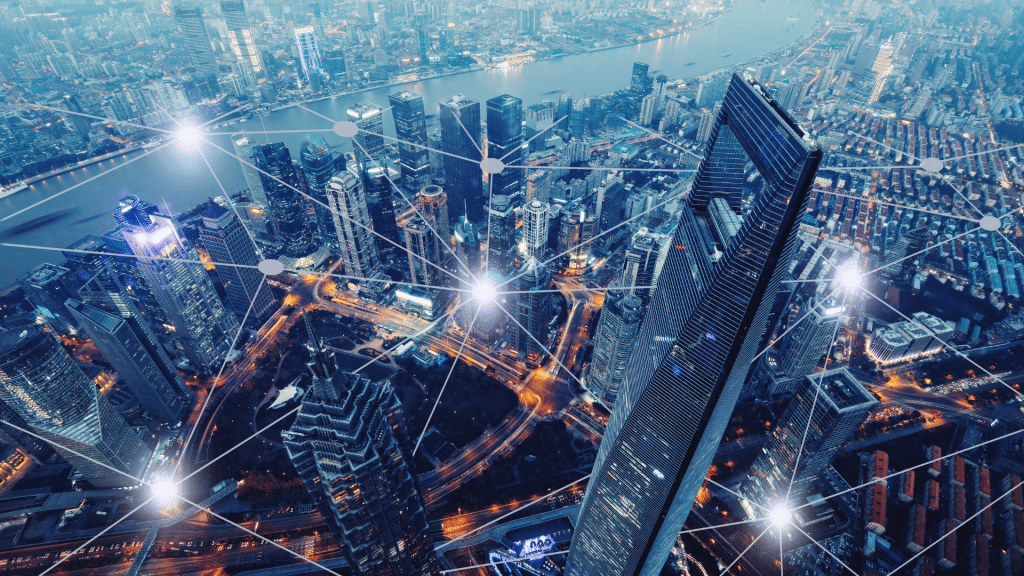
By Jeff Geiser, VP of Customer Experience at Zenlayer
Where is the edge? That question has popped up in a bunch of recent meetings as we talk about cloud computing and its ‘long haired, hippy’ sibling edge computing. Even for us techie types the edge can be difficult to pin down. Roughly 10 years ago, Cisco introduced the term “fog computing” – and given all the choices that exist to place compute these days, finding the edge is more like playing hide and seek in the mist than ever.
The edge will always vary for different use cases, so to describe the edge, let’s borrow a leaf from John Gage’s 80’s playbook. While at Sun Microsystems, he coined the phrase “the network is the computer.” John was describing access to compute that existed out on the network beyond workstations. In a slight adaptation, I would like to propose “the network is the edge.”
Maybe more specifically, every network transition your data zips through has the potential to be the edge. So, where should you put your edge? While data privacy and management of the infrastructure are always factors that could sway the outcome, the cost and relative importance of latency for your application is the key factor driving the decision.
Take cars, for instance. They have cameras or LiDar generating a high volume of data that a neural network consumes in order to make split-second driving decisions. The inference model needs to perform the forward propagation within milliseconds – very low latency. Many of these “embedded edge” or “product edge” devices either generate large volumes of data that can be difficult to transfer to another location quickly enough or it is simply cheap enough to make these decisions on the local device. If you need at-source, low latency decisioning or visualization then the embedded edge will be the first edge to consider.
Devices and applications that still need low latency but can accommodate the time introduced by sending data upstream another hop or two may leverage an edge in the telco providers network. For example, maybe a smart city device leverages compute at a 5G MEC in order to determine an action. The 5G MEC provides low latency connections and allows for some of the compute logic and decisioning to reside beyond the embedded edge in order to be scaled across a large number of devices. This “mobile edge” is generally where the fog edge begins – the fog of edge variations that precede the hyperscaler cloud edge we most commonly think of today.
This is where the “fog edge” typically begins. A combination of edge choices that might be a private enterprise cloud or datacenter, telco cloud, internet data center or exchange – really any variation of edges that precede the hyperscaler cloud edge that is most often thought of today. Functions that evaluate and manipulate the flow of data might be deployed in a CDN edge that is a network hop or two from the local ISP. Inference models, games, and real-time financial applications might allow for compute to be deployed at a well-connected peering exchange or meet-me datacenter that has a unique blend of IP transit and peering. Training a large language model might require GPU deployment in a core data center or a hyperscaler. Depending on the latency requirements, we can evaluate the overall network path from devices, data, and users and find a network edge that provides the best balance between latency and cost for the application.
Willner, Alexander & Gowtham, Varun. (2020). Towards a Reference Architecture Model for Industrial Edge Computing.
In the evolving landscape of edges, businesses are increasingly turning to a range of solutions to bolster their strategic initiatives and achieve their business objectives. By leveraging different edge environments, companies can ensure that they are optimizing their operations for efficiency, scalability, and security. This multi-edge approach allows for optimizing that balance between latency and cost, as well as the agility to adapt to market demands and technological advancements. As a result, choosing an edge provider that can accommodate a wide array of use cases has become complicated.
Zenlayer’s hyperconnected cloud is deployed in the fastest-growing and the hardest-to-reach markets and we strive to provide the lowest latency edge for applications.
The company has over 280 PoPs that are hyperconnected to local networks and also have access to private backbones for low latency connectivity to other cities or regions. In zenConsole, you can evaluate latency between our PoPs and end-user networks to assess the best location for bare metal or virtual machine deployment. It’s also used to evaluate the latency between PoPs and deploy a layer 2 point-to-point or fully meshed layer 3 connection between PoPs in other cities or regions.
Each PoP has a BGP blend with a wide range of local peers and transit providers in order to optimize traffic for Zenlayer’s customers.
As technology evolves and the edge expands, choosing a partner that can accommodate a wide range of compute and low latency options is critical.
About the author
Jeff Geiser comes with over 15 years of experience in technical leadership at startups and distinguished technology companies like UUNet, Akamai, and most recently, EdgeCast (now Edgio), where he led teams responsible for the design, production, onboarding, and deployment of solutions for notable clients that included Twitter, Disney/ESPN, and PayPal. In his capacity as the VP of Customer Experience at Zenlayer, Jeff leads Zenlayer’s technical support team to ensure an unparalleled level of service regardless of where customers choose to deploy.
DISCLAIMER: Guest posts are submitted content. The views expressed in this post are that of the author, and don’t necessarily reflect the views of Edge Industry Review (EdgeIR.com).
Article Topics
CDN | edge computing | fog computing | PoP | Zenlayer
Comments