Letting IoT edge devices decide how to grab some 5G spectrum
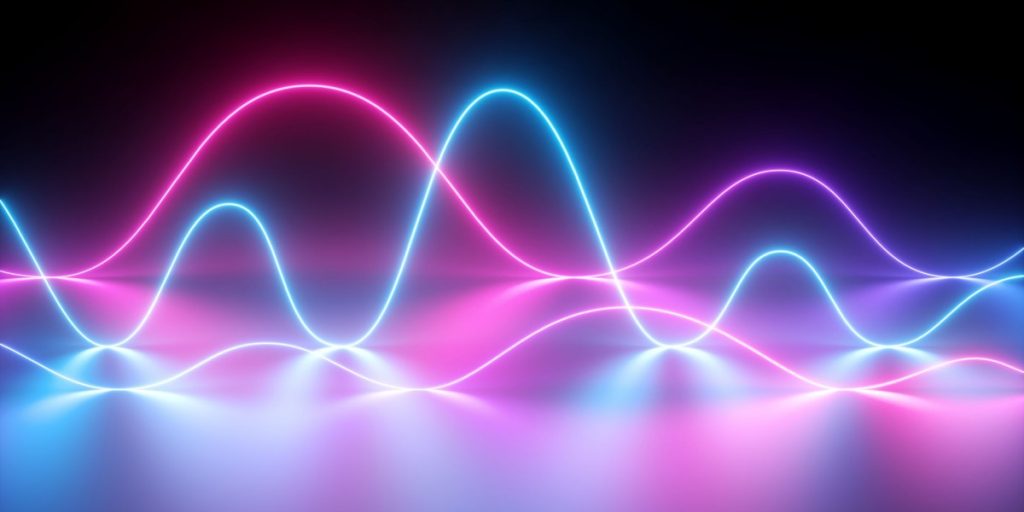
The cacophony of signals generated by cellular IoT devices threatens to slow or destabilize the Internet of Things long before it can become ubiquitous. Two researchers at Northeastern University in Boston think they can bring order to the digital babble by putting deep-learning algorithms within wirelessly connected edge hardware, making the devices adaptive and resilient.
For the most part, complex, hard-coded mathematical models in IoT devices can predict traffic patterns, noise, interference, and other corrupting properties of spectrum overuse, even in what passes for hectic environments today. So armed, the devices can grab unused spectrum snippets on the fly on which to send their signals.
But the increased density of devices, whether in a large factory or at a busy address in Manhattan, is creating radio traffic that grows and grows. In some instances, the volume already is beyond the detangling power of preset communication scenarios.
Solving the radio problem with on-device deep learning
Francesco Restaucci and Tommaso Melodia are part of the Northeastern University’s Institute for the Wireless Internet of Things. The researchers propose using “cognitive radios” that provide real-time and effective “fine-grained optimization of spectrum resources.”
Theirs is called a spectrum-driven approach as opposed to a model-driven approach (discussed above) and parochial protocol-driven approach, which is defined by the communication protocol (such as WiFi) used for signaling.
“The big picture is to realize systems able to distinguish on their own different spectrum states … and change their hardware and software fabric in real-time,” the pair wrote. Doing so will require implementing real-time deep learning on the device.
They say the idea of cognitive radio is more than two decades old, but the computing power needed to deliver it has not been practical, and even now presents challenges. Deep learning models will require the real-time processing of hundreds of megabytes of data per second.
The report’s authors suggest a need for “wireless data factories” to process waveform data and tune the radio to an optimal, available frequency.
Comments