How compute-in-memory can power edge AI and accelerate manufacturing efficiency
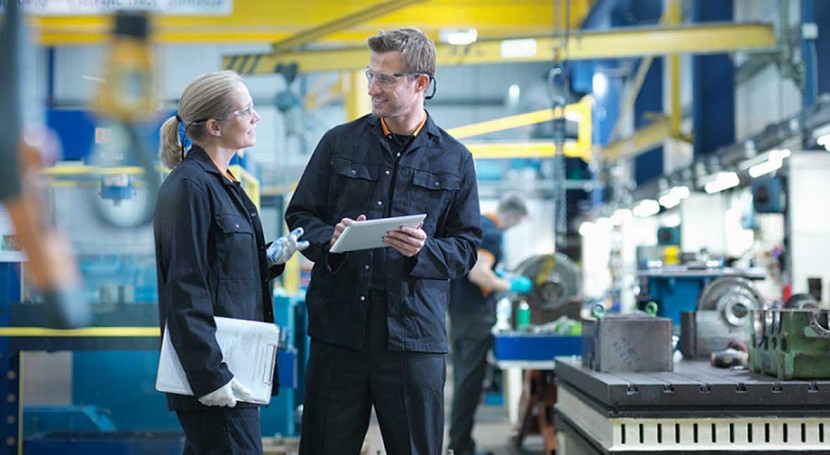
By David Kuo, Senior Director Business Development and Product Marketing at Mythic Inc
Factories have made significant advancements in efficiency during the past few decades; increasingly, they are using smart robots and other connected machines to increase production efficiency. Now the next wave of transformation for the manufacturing industry is coming thanks to advancements in edge AI processing, allowing factories to combine the capabilities of computer vision (CV) with AI to boost production volumes and manufacture things more quickly, efficiently and cost-effectively.
One example of this progression is how factories are deploying artificial intelligence (AI) and deep learning (DL) solutions to inspect products on the production line in real time, which includes everything from inspecting cereal boxes for defects to examining cars for scratches. Not only can machines detect anomalies on the production line more accurately, AI is also enabling them to make intelligent decisions in real time. This is made possible by extremely powerful edge AI solutions that no longer have to send data to the cloud for processing, but can now accurately process huge volumes of information, with extremely low latency, from deep neural network (DNN) at the edge.
The promise of compute-in-memory
One approach that has shown incredible potential is analog compute-in-memory (CIM). AI processing at the edge requires silicon that can meet demanding requirements for small form factor, low latency, and low power consumption, and it’s becoming increasingly clear that these requirements have outpaced the capabilities of digital solutions like CPUs and GPUs. This new approach of utilizing analog compute in flash memory provides several key benefits.
First, CIM is amazingly efficient; by leveraging the flash memory element for both neural network weight storage and computation, it eliminates data movement between system components during AI inference processing. This leads to high performance and low latency while dramatically increasing power efficiency for inference operations. CIM is suitable for computing the hundreds of thousands of multiply-accumulate operations occurring in parallel during vector operations. This type of low-latency, accurate processing is absolutely essential in the industrial sector where thousands of products are being manufactured every minute and real-time detection is critical to manufacturing throughput.
Additionally, the analog CIM approach takes advantage of high-density flash memory to enable compact, single-chip processor designs. This allows analog CIM processors to go into machines of all sizes, whether it’s a small drone doing safety inspections or a machine vision system in a production line. Furthermore, analog CIM processors are produced with mature semiconductor process nodes with much better supply chain availability and lower device cost when compared to digital solutions that use bleeding-edge semiconductor process nodes.
Perhaps most importantly, analog CIM dramatically reduces power consumption, making it 10X more efficient than digital systems while still enabling extremely high-performance computing. Analog computing is the ideal approach for AI processing because of its ability to operate using much less power at a higher performance with millisecond latencies. The extreme power efficiency of analog compute technology will let product designers unlock incredibly powerful new features in small edge devices, and will help reduce costs and a significant amount of wasted energy in all types of AI-based manufacturing applications.
As the need for automation in factories continues to grow, more powerful AI and deep learning capabilities in certain processors will improve the accuracy and efficiency of day-to-day operations. This opens the door to introduce even more “smart” applications into today’s factories, from cobots to autonomous delivery drones to self-driving forklifts and beyond. Without the existing limitations on power, cost and performance, the possibility for innovation is endless.
About the author
David Kuo is the Senior Director of Product Marketing and Business Development at Mythic Inc, a manufacturer of high-performance and low-power AI accelerator solutions for edge AI applications.
DISCLAIMER: Guest posts are submitted content. The views expressed in this post are that of the author, and don’t necessarily reflect the views of Edge Industry Review (EdgeIR.com).
Cvedia to use Hailo AI chips for “thermal edge” for Smart City, transportation monitoring
What is edge AI and what is it used for?
Article Topics
chip | CIM | computer vision | edge AI | Industry 4.0 | manufacturing | Mythic Computing | neural network
Comments